Table of Contents
show
Simple Feature Scaling
Divides each value by the maximum value of that feature,
#To Normalize one column: df['col_name']=df['col_name'] / df['col_name'].max()
Example:
df=df['length'] = df['length'] / df['length'].max()
df
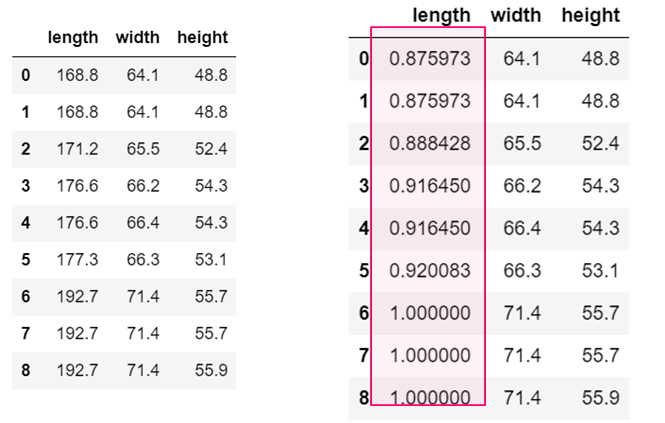
Normalization for length attributes,
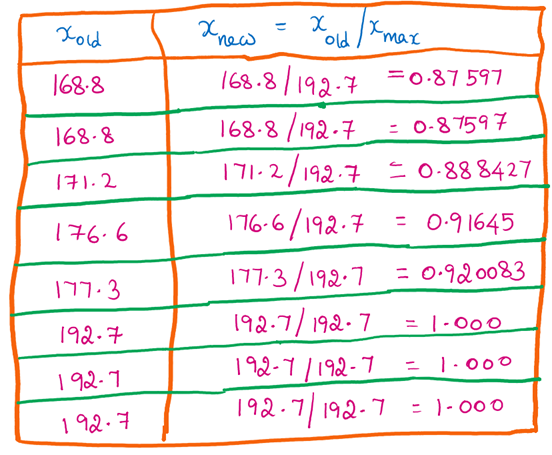
Formula:
\[x_{new} = {x_{old} \over x_{max}}\]
To Normalize the entire dataset
df2 = df / df.max()
df2
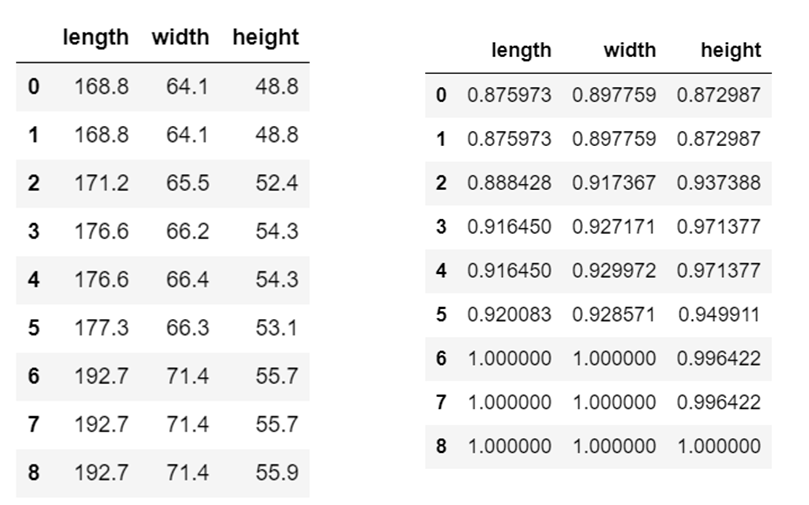
Min Max Normalization
# To normalize one column:
df['col_name'] = (df['col_name'] - df['col_name'].min()) / (df['col_name'].max() - df['col_name'].min())
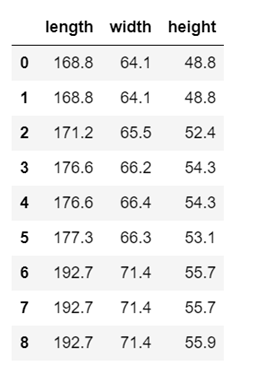
Take each value, subtract it from minimum value of that feature then divides by the range of that feature,
Formula:
\[x_{new} = {x_{old} – x_{min}\over x_{max} – x_{min}}\]
df_mmn['length’]= (df_mmn['length']-df_mmn['length'].min()) / (df_mmn['length'].max() - df_mmn['length'].min())
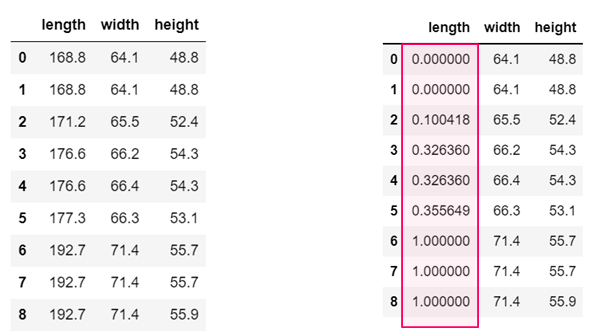
Example:
Normalization of length attributes,
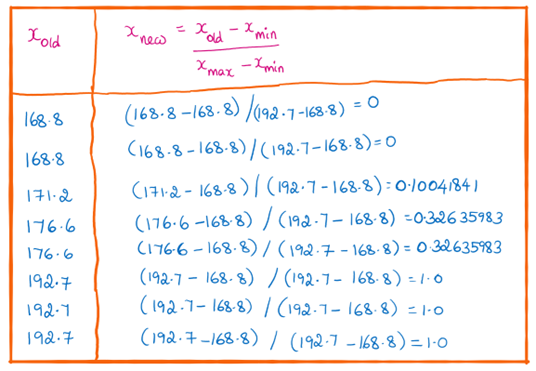
#To normalize entire dataset:
Syntax:
df = (df-df.min()) / (df.max() - df.min())
Example:
df_mmn = (df_mmn - df_mmn.min()) / (df_mmn.max()-df_mmn.min())
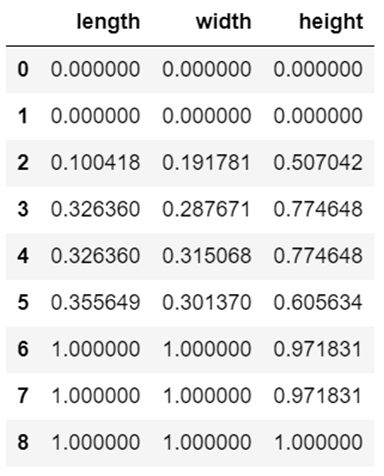
Z – Score Normalization
# To normalize one column:
df['col_name'] = (df['col_name'] - df['col_name'].min()) / (df['col_name'].max() - df['col_name'].min())
For each value, subtract the mean of the feature and then divide by standard deviation
Formula
\[x_{new} = {x_{old} – \mu\over \sigma}\]
\[\mu\]
\[\]
\[\sigma\]
represents Mean
represents Standard Deviation
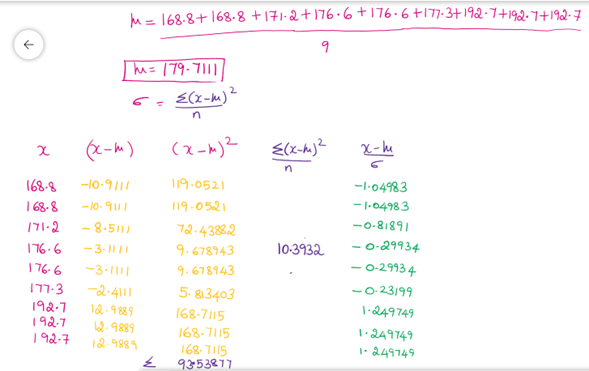
df_zs['length'] = (df_zs['length'] -df_zs['length'].mean()) / df_zs['length'].std()
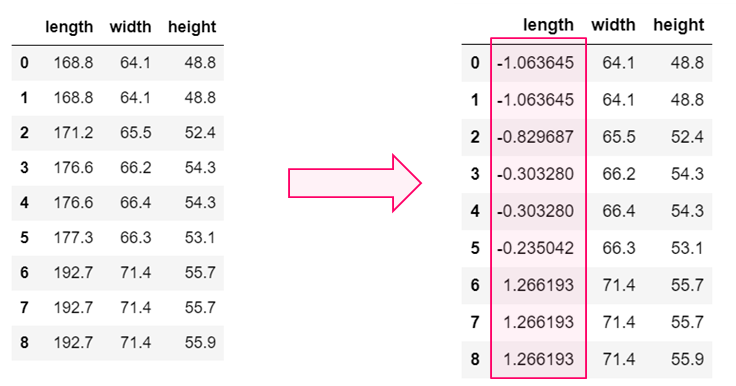
df_zs = (df_zs - df_zs.mean()) / df_zs.std()
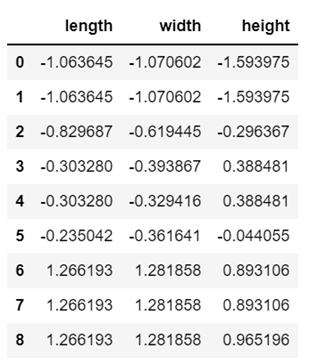
Views: 2